Mission Statement
The core research topics of the Computational Science Center are Inverse Problems and Image Analysis. The common thread among these areas is a canonical problem of recovery of an object (function or image) from partial or indirect information. Particular research topics are:
- Geometrical Modeling for Image Analysis.
- Mathematical Modeling and numerical simulations in Coupled Physics Imaging.
- Variational regularization methods for Image Analysis and Inverse Problems.
- Mathematical models for visual attention.
News
Professor Scherzer has been nominated for his prolific and profound research in the field of inverse and ill-posed problems, with breakthrough results in regularization theory, tomography, and the mathematics of imaging. This is the latest in a long line of awards and recognitions, including the Förderungspreis of the Austrian Mathematical Society, the START Prize of the Austrian Science Fund, the Prize of the Tyrolean Science Foundation and the Distinguished Scientist Award of IPIA, to name a few. Congratulations, Otmar!
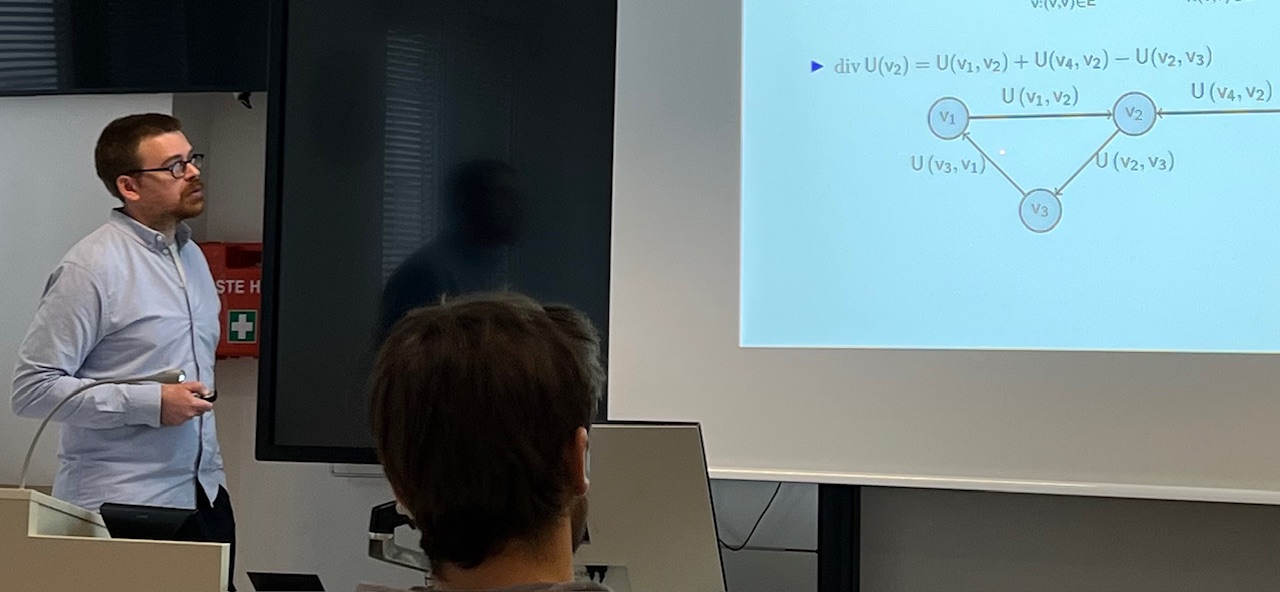
We are delighted to announce that Clemens Kirisits has successfully defended his Habilitation thesis! On March 19th, 2025, Clemens presented his work titled “Total Variation Denoising with ℓ¹ Anisotropy – Approximation, Convergence Rates and Universal Minimality”, marking the culmination of years of dedication and hard work.
On March 19th, 2025 we welcome our guest Liliana Borcea. She will give a talk — Data driven reduced order modeling for first order hyperbolic systems with application to waveform inversion — at Universität Wien, BZ02, Oskar-Morgenstern Platz 1, 1090 Wien. 13:00 Vienna Time
Abstract: Waveform inversion seeks to estimate an inaccessible heterogeneous medium by using sensors to probe the medium with signals and measure the generated waves. It is an inverse problem for a hyperbolic system of equations, with the sensor excitation modeled as a forcing term and the heterogeneous medium described by unknown, variable coefficients. The traditional formulation of the inverse problem, called full waveform inversion (FWI), estimates the unknown coefficients via nonlinear least squares data fitting. For typical band limited and high frequency data, the data fitting objective function has spurious local minima near and far from the true coefficients. This is why FWI implemented with gradient based optimization can fail, even for good initial guesses. We propose a different approach to waveform inversion: First, use the data to ``learn” a good algebraic model, called a reduced order model (ROM), of how the waves propagate in the unknown medium. Second, use the ROM to obtain a good approximation of the wave field inside the medium. Third, use this approximation to solve the inverse problem. I will give a derivation of such a ROM for a general first order hyperbolic system satisfied by all linear waves in lossless media (sound, electromagnetic or elastic). I will describe the properties of the ROM and will use it to solve the inverse problem for sound waves.
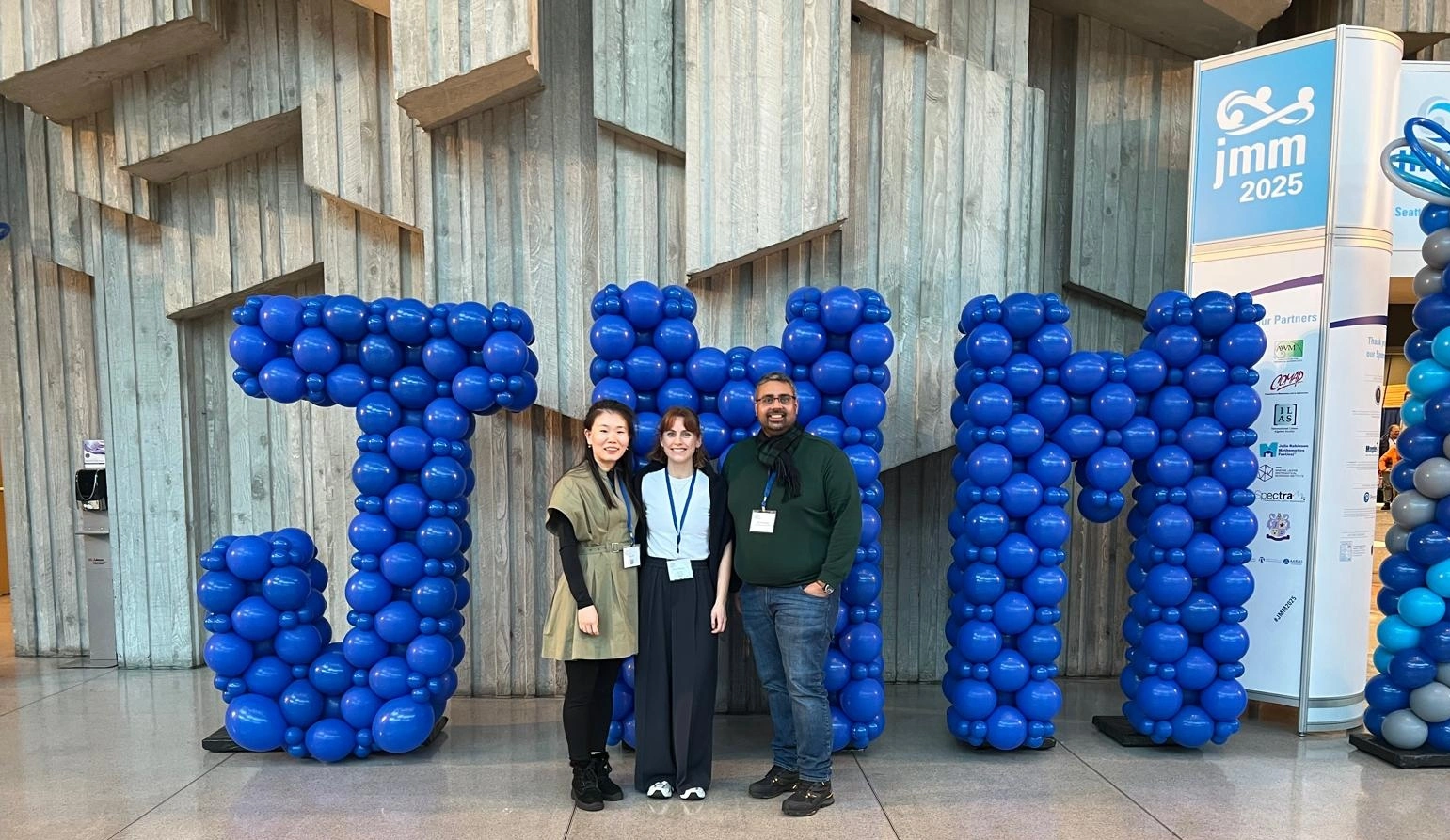
From January 8 to 11, 2025, the largest mathematics gathering in the world, JMM 2025, brought together thousands of researchers, educators, and students in Seattle, USA. Hosted by the American Mathematical Society (AMS) in collaboration with 16 partnering organizations, the conference offered a unique platform for sharing knowledge and fostering collaboration across diverse fields of mathematics.
As part of the AMS Special Session on 'Recent Developments in Regularization Methods for Nonlinear Inverse Problems', co-organized by Otmar Scherzer, our team members Cong Shi, Kamran Sadiq, and Noemi Naujoks were invited to present their newest results.
We are honored to have been part of this event and are grateful for the opportunity to connect with the global mathematics community.